Alright, let’s talk about this “yoshihito nishioka prediction” thing I messed around with today. It was a bit of a rabbit hole, but I learned a few things, so figured I’d share.
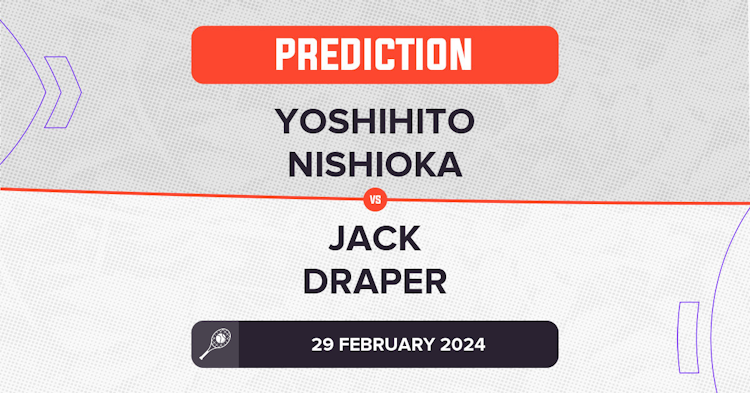
So, the basic idea was, could I build a simple model to predict the outcome of Yoshihito Nishioka’s tennis matches? I know, I know, probably a fool’s errand, but hey, gotta try, right?
Data Gathering:
First things first, I needed data. I started by scraping match results from a tennis stats website. Found one that had a decent amount of historical data on Nishioka, like match scores, opponents, tournament types, and even some surface information (hard, clay, grass, etc.). The scraping part was a pain. The website wasn’t exactly designed for easy data extraction, so I had to write some pretty clunky Python scripts using Beautiful Soup to navigate the HTML and pull out the relevant bits.
After scraping, I ended up with a CSV file with a bunch of rows, each representing a match. Columns included:
- Date
- Opponent
- Result (Win/Loss)
- Tournament
- Surface
- Score (e.g., 6-4, 7-5)
Data Cleaning and Feature Engineering:
Okay, now the fun part – cleaning up the mess. The raw data was, as expected, pretty dirty. Dates were in inconsistent formats, opponent names had typos, and the scores were just strings I needed to parse. I used Pandas in Python to wrangle this. Converted dates to a standard format, cleaned up opponent names (best I could), and split the score strings into sets won by each player.
Then came the feature engineering. I figured just feeding the raw data into a model wouldn’t be enough. I needed to create some more informative features. Here’s what I came up with:
- Nishioka Win Percentage (Last 10 Matches): A rolling average of his wins over the previous 10 matches. I hoped this would capture his recent form.
- Opponent Win Percentage (Last 10 Matches): Same as above, but for his opponent.
- Surface Type (One-Hot Encoded): Created separate columns for each surface type (Hard, Clay, Grass) and set them to 1 or 0 depending on the surface of the match.
Model Building:
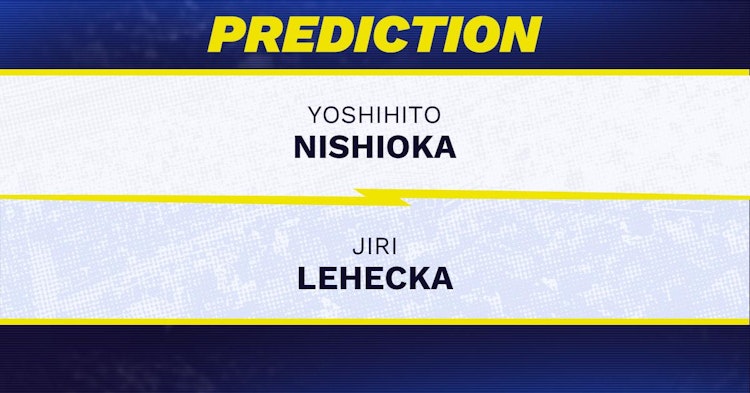
For the model itself, I decided to keep it simple and use a Logistic Regression. I used scikit-learn in Python. I split the data into training (80%) and testing (20%) sets. Trained the Logistic Regression model on the training data, using the features I engineered to predict the “Result” (Win/Loss).
Evaluation:
Alright, time to see how garbage my model was. I used the test data to make predictions and then calculated the accuracy. The accuracy was… not great. Something like 62%. Better than a coin flip, but not by much.
What I learned:
This whole thing was a good reminder that predicting sports outcomes is hard! There are so many factors that I didn’t even begin to account for. Things like player fatigue, injuries, mental state, and even weather conditions can all play a huge role.
Also, my data was pretty limited. I only had a few years of Nishioka’s match data, which isn’t really enough to train a robust model. And the features I engineered were pretty basic. More sophisticated features, like player rankings, Elo ratings, or even betting odds, might have improved the results.
Overall, it was a fun little project. I got to practice my data scraping, cleaning, and machine learning skills. And who knows, maybe with more data and better features, I could actually build a decent tennis prediction model someday. But for now, I’ll just stick to watching the matches and enjoying the sport!